Mastering Moving Average Calculations for Success
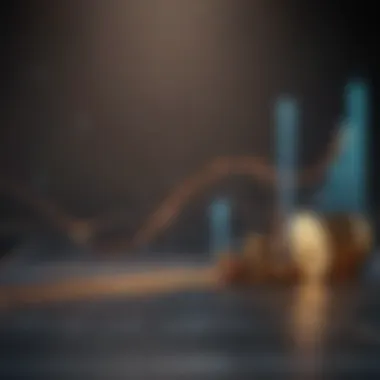
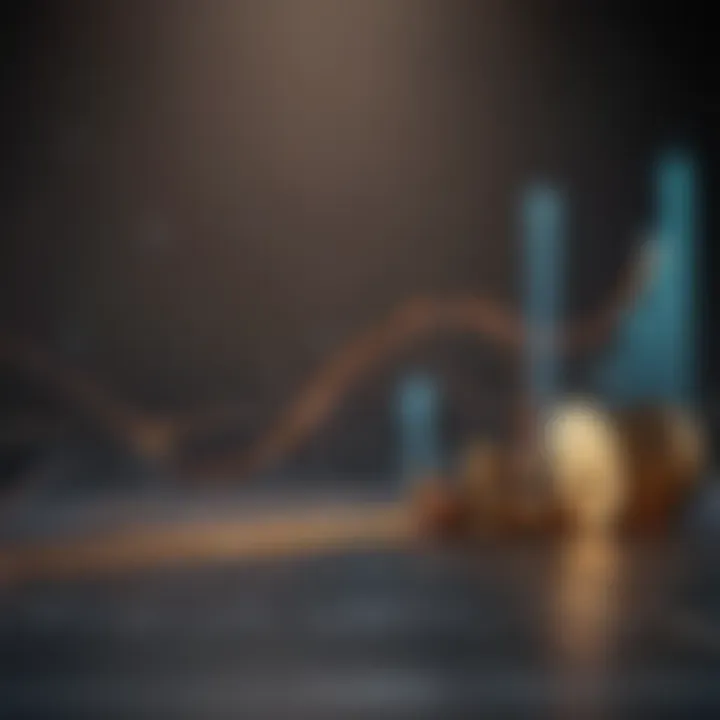
Investment Products Overview
Investment products are essentially the vehicles through which individuals and institutions seek to grow their wealth. They come in many shapes and sizes, each offering different levels of risk, potential returns, and liquidity. Understanding these products is crucial for anyone looking to dip their toes into the world of finance.
Definition of Investment Products
Investment products can be described as financial instruments or vehicles that allow investors to allocate their resources in a way that they aim at achieving a certain level of financial return. These can span from stocks and bonds to mutual funds and real estate.
This definition encompasses a wide array of options, catering to varying risk tolerances and investment goals. While one product might dive headfirst into high-risk avenues, others may prioritize stability and steady income.
Types of Investment Products
The spectrum of investment products available can often feel overwhelming, but here are some of the most common categories:
- Stocks: Purchasing shares of a company can lead to substantial profits, but also comes with the risk of loss.
- Bonds: Considered a safer option than stocks, bonds typically represent loans made to governments or corporations.
- Mutual Funds: These are pooled resources from multiple investors, allowing for diversification across stocks, bonds, and other securities.
- Exchange-Traded Funds (ETFs): Similar to mutual funds but traded like stocks throughout the day, ETFs often carry lower fees and are quite flexible.
- Real Estate Investment Trusts (REITs): For those who wish to invest in property without the hassle of direct ownership, REITs provide a nifty way to enjoy real estate profits.
In each category above, investors can also consider the weight of moving averages, which can provide invaluable insights into performance and market trends.
Comprehensive Investment Dictionary
Investment terminology can at times be a labyrinth, especially for those who are just getting their feet wet. Defining key terms is essential for proper understanding and engagement with the investment landscape.
Key Terms Explained
- Moving Average: A statistical calculation that analyzes data points by creating averages of different subsets of the complete data set, often used in stock analysis.
- Volatility: Refers to the degree of variation in trading prices over time, a vital indicator of risk.
Glossary of Financial Terms
An insightful glossary of terms could benefit many investors. Here are a few that are worth keeping in mind:
- Liquidity: The ease with which an asset can be converted into cash without affecting its market price.
- Diversification: The practice of spreading investments across different assets to reduce risk.
- Capital Gains: Earnings from the sale of a capital asset, such as stocks and bonds, that have increased in value.
"In the investment world, understanding terminology is as critical as understanding the products themselves. A small misstep can lead to large financial repercussions."
Getting to grips with these terms is half the battle when delving into the realm of investments, particularly as you start exploring the principles underlying moving averages and their implications in decision-making.
Preamble to Moving Averages
The concept of moving averages is essential in many fields, especially finance, and data analysis. Understanding moving averages can help individuals and organizations make better decisions based on historical trends and future projections. Rather than simply viewing raw data, moving averages provide a clearer picture by smoothing out fluctuations, allowing practitioners to focus on the overall trend rather than the noise.
Definition of Moving Average
In its essence, a moving average is a statistical calculation that takes a set of values from a dataset and averages them over a specific time period. This can either be over days, months, or even years, depending on the context in which it is being applied. By continuously updating the dataset to calculate the average, it becomes a dynamic measure that reflects the ongoing changes within the dataset.
The most straightforward example would be a simple moving average where you take the arithmetic mean of a specific number of data points, say, the last five days of stock prices, and then shift that window forward one day at a time. This allows analysts to see trends that can be hidden in day-to-day fluctuations.
Importance in Data Analysis
Moving averages are a cornerstone of data analysis because they help analysts and investors interpret trends in a more digestible way. For example, in stock trading, a moving average can signal whether a stock is in an uptrend or downtrend. Studying these trends provides valuable insights when making crucial economic decisions.
- Highlighted Benefits of Using Moving Averages:
- Reduces noise in data, making it easier to identify trends.
- Provides a clearer view of potential future movements based on historical data.
- Serves as a fundamental component in various trading strategies.
Thus, the significance of moving averages in data analysis transcends mere calculation; they facilitate comprehension of complex datasets, leading to informed decision-making.
Applications Across Disciplines
Moving averages find their utility across various fields, laying down a framework that is both versatile and impactful. Here are a few notable applications:
- Finance: Investors and traders rely on moving averages to identify entry and exit points in the market.
- Economics: They help in analyzing economic indicators such as GDP growth over time, smoothing volatile economic data for broader insights.
- Healthcare: In public health data, moving averages can be used to track the spread of diseases, providing a clearer view of trends in infection rates over time.
- Manufacturing: They can also help in quality control, where consistent measurements over time may indicate production process issues.
Ultimately, moving averages emphasize the universality of this statistical tool. While the calculation may seem straightforward, its applications are profound and diverse. Understanding them opens up avenues in many professional realms and everyday decision-making.
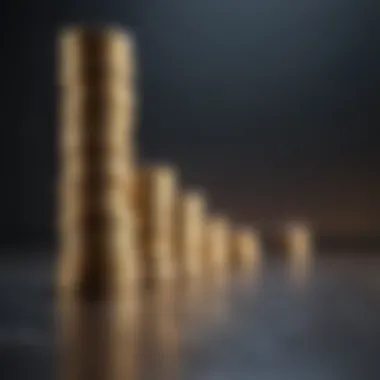
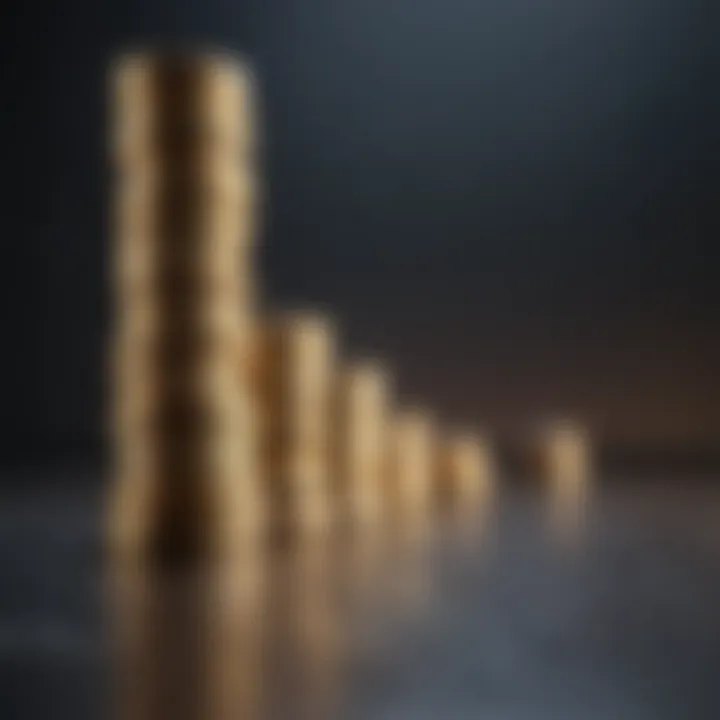
Types of Moving Averages
When diving into the world of moving averages, it's crucial to grasp the array of types available. They offer differing perspectives on data trends and behaviors, which can't be overstated. Each type presents its own flavor, addressing unique analytical needs while providing insightful depth. Understanding moving averages isn't just an academic exercise; it’s about employing the right tool for the job.
Simple Moving Average
Calculation Methodology
The simple moving average (SMA) is a foundational technique in financial analysis. The calculation involves summing the values over a specified period and dividing by the number of periods. For example, if you're examining the stock prices for the last five days, you would add those prices and then divide by five. One key characteristic of the SMA is its straightforwardness, making it a friendly choice for beginners.
This simplicity is its strength and also its weakness. While easy to compute, the SMA can lag behind current trends since it treats all data points equally. Hence, its unresponsiveness to sudden price changes can lead to missed opportunities or delayed reactions, particularly crucial in fast-moving markets.
Advantages and Limitations
The SMA serves as a powerful indicator of overall market direction. Its clarity and ease of calculation makes it popular among novice traders who may not have advanced statistical knowledge. However, the unique feature of displaying equal weight to each data point can overly simplify more complex market scenarios, leading to misinterpretations. Moreover, when market volatility spikes, the lagging nature becomes pronounced, potentially skewing trading decisions.
Weighted Moving Average
How It Differs from Simple Moving Average
The weighted moving average (WMA) shifts the focus from simplicity to accuracy. Unlike the SMA, which treats all data points the same, the WMA assigns different weights to each observation, usually giving more importance to recent data. This characteristic makes it a beneficial strategy when real-time, responsive analytics are necessary. The process of assigning weights introduces a necessary nuance that the simple version lacks, aligning better with rapid changes in trading environments.
As it emphasizes recent values, active traders often prefer the WMA for short-term investments. However, care needs to be taken when determining weights, as arbitrary choices can misrepresent trends instead of clarifying them.
Use Cases in Financial Markets
Financial analysts frequently utilize the WMA in assessing stock trends, especially in volatile markets. Its ability to react more swiftly to price shifts grants traders crucial insights when timing their investments. The unique feature of pubishing recent data as more relevant is key here; it allows for more refined gauges of market conditions compared to the SMA. Nonetheless, investors should remain cognizant of the risk involved; incorrect weight assignments can complicate market interpretations rather than simplify them.
Exponential Moving Average
Calculation and Characteristics
The exponential moving average (EMA) takes the weighted approach one step further. In the EMA, math holds a profound allure—it assigns weights in an exponentially decreasing manner as the prices go further back in time. This approach means that latest prices are more heavily weighted than prior prices. The implication of this calculation is that EMA provides a fine-tuned reaction to market shifts, an asset for traders interpreting fickle conditions.
Its appeal lies in both speed and sensitivity, making it a favored tool for tech-savvy traders who appreciate real-time data reactions. However, its higher sensitivity may introduce volatility, leading to potentially misleading signals in choppy market conditions.
Implications for Trading Strategies
For trading strategies, the EMA serves as an essential instrument. Many traders pair it with other indicators to enhance their strategies through confirmation—ensuring that a potential trade is backed by another signal. The ability to gauge momentum swiftly helps in decision-making processes, related to buy and sell orders. Still, a word of caution is warranted: the EMA’s sensitivity can sometimes lead to overtrading, as one can chase market noise rather than genuine signals.
Calculating Moving Averages
Calculating moving averages is a cornerstone in various analytical fields, particularly in finance. This systematic approach smooths out fluctuations in data, providing a clearer view of trends over time. It’s like putting on a pair of glasses that helps you see beyond the daily chaos, revealing the underlying patterns that matter. This section serves to demystify the process, ensuring you’re well-equipped to tackle moving averages, whether you're a seasoned investor or just dipping your toes in the water.
Step-by-Step Guide for Simple Moving Average
Data Collection
The foundation of calculating a simple moving average lies in solid data collection. Without accurate and relevant data, your analysis can become as reliable as a weather forecast in a snowstorm. Data collection for moving averages typically involves gathering numerical values over a specific time frame, like daily stock prices.
The essential characteristic of data collection here is its focus on responsiveness to changes. By ensuring that your data includes the most recent values, you establish an up-to-date backdrop for your analysis. This choice is not just beneficial but critical in the fast-paced world of finance, where every tick matters.
However, a unique challenge comes with data collection: ensuring consistency. Inconsistent data can skew your results, leading to potential misinterpretations. A systematic approach to collecting data can save you from future headaches and enhance the accuracy of your moving average calculations.
Performing the Calculation
Now that you've got your data, it's time to perform the calculation. This step is like mixing the right ingredients to bake a cake; you want to ensure the proportions are correct. Calculating a simple moving average involves summing the selected data points and dividing them by the number of data points. For example, if you were analyzing five days of stock prices, you would add those prices together and divide by five.
The key characteristic of performing this calculation is its simplicity. What makes this method popular is its straightforwardness. There's little room for error if done correctly, a comfort in any calculation. However, its simplicity can also be a double-edged sword. While easy to calculate, a simple moving average can be slow to adapt to rapid changes in trends, which is a consideration that shouldn’t be overlooked.
Weighted Moving Average Calculation
Assigning Weights
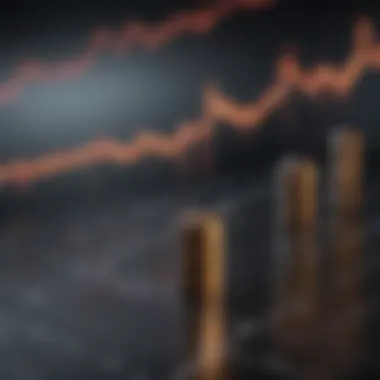
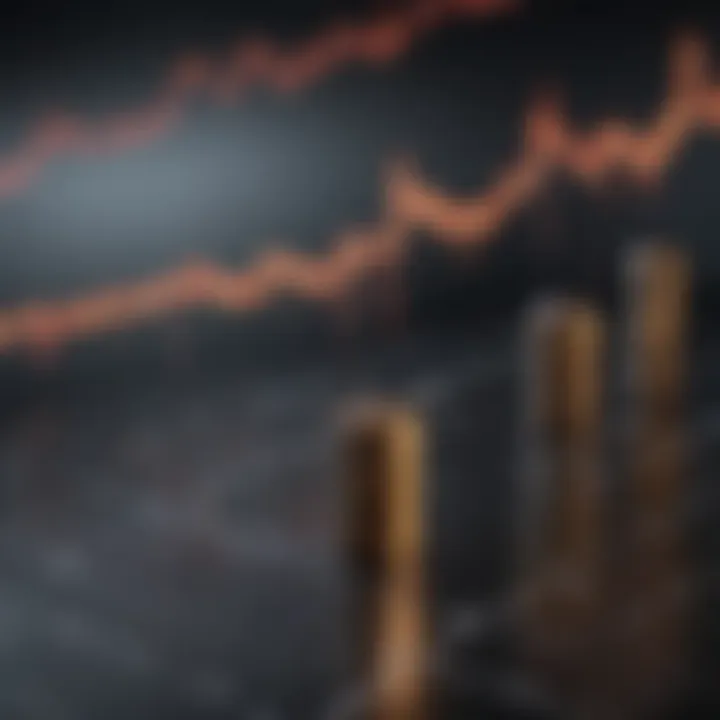
The weighted moving average introduces a depth to your analysis that a simple moving average may lack. Assigning weights allows for a more nuanced view by emphasizing recent data over older data. In this approach, you might assign a weight of 3 to the most recent day, a weight of 2 to the previous day, and a weight of 1 to the last day, for instance. This way, your calculations reflect the importance of new information.
This method is beneficial as it enhances the relevance of your analysis, aligning it closer with real-time market shifts. A unique feature here is that the weight can be customized depending on the investor's strategy. But be cautious: overemphasizing recent data can create a snapshot that dances to the latest tune but misses the bigger picture.
Final Calculation Process
Engaging in the final calculation process for weighted moving averages is like crafting a piece of fine art. After assigning weights, you take the data points, multiply each by its corresponding weight, and sum them up. Finally, divide by the total weights assigned. This meticulousness allows for a more tailored analysis.
The process gains appeal as it captures dynamic changes in data better than its simpler cousin. Just like a tailored suit, the weighted moving average fits better to the individual investor’s approach. However, it can be more complex to execute, demanding closer attention to detail.
Exponential Moving Average Calculation
Understanding the Smoothing Factor
When diving into the exponential moving average (EMA), you encounter the smoothing factor, which adds another layer of finesse to your analysis. This factor determines how much weight is placed on the most recent price in your calculations. A proper understanding of this smoothing factor can make or break the effectiveness of your EMA.
What makes the smoothing factor appealing is its adaptability. By adjusting it, you can tailor your analyses to either respond swiftly to price changes or filter out noise. This flexibility is invaluable for traders who need to navigate a rapidly changing environment. However, if set improperly, it may lead to overstating minor fluctuations instead of addressing larger trends.
Calculating Step-by-Step
Finally, we come to the step-by-step calculation for the exponential moving average. Start by calculating the weighting multiplier, which is typically expressed as 2 divided by the number of periods plus one. Multiply this factor by the price difference between the current price and the previous EMA value, then add the previous EMA.
This method is beneficial due to its responsiveness to new data, providing investors with a timely indicator of trends. The unique feature here is that the EMA places greater emphasis on the most current data points, which can help in making informed trading decisions. Still, it requires a solid understanding of its methodology—missteps can lead to confusion in trend interpretation.
Practical Applications of Moving Averages
Moving averages are not just figures on a chart; they’re essential tools that help make sense of market noise and provide clarity to data trends. The practical applications of moving averages span across various domains, but they shine particularly bright in finance, where they influence trading strategies, market analysis, and economic forecasting. Understanding how to apply them effectively can empower investors and analysts alike to navigate through uncertainty with informed precision.
Using Moving Averages in Stock Trading
Signal Generation
Signal generation is one of the cornerstone benefits that moving averages bring to stock trading. By smoothing out price data, they create a clearer picture of market movements. Traders often look for signals, which are points in time that indicate when to buy or sell. For instance, when a short-term moving average crosses above a long-term moving average, it generates a buy signal, suggesting an upward trend. Conversely, a cross in the opposite direction triggers a sell signal.
The key characteristic of signal generation lies in its timing and accuracy. Many traders prefer it because it helps in filtering out market volatility by focusing on sustained trends rather than erratic price swings. However, like any method, it isn’t without drawbacks. The phenomenon known as lagging can cause traders to enter or exit positions later than ideal, potentially missing the best moment.
Market Trend Analysis
Market trend analysis is another area where moving averages come in handy. This analytical approach utilizes moving averages to identify overall market direction. With a visual representation, it’s easier to determine bullish or bearish trends. Traders can even compare multiple moving averages over different periods to gauge momentum and reversals.
The uniqueness of this technique lies in its power to reveal underlying market sentiments with simplicity. It’s popular because it sidesteps excessive noise and focuses on consistent data. However, it’s also essential to recognize that relying solely on this method might lead one to overlook sudden market shifts, which can potentially derail trading plans.
Moving Averages in Economic Indicators
Analyzing Economic Cycles
In economic landscapes, moving averages play a crucial role in analyzing economic cycles. By smoothing economic data, such as GDP growth rates or unemployment figures, analysts can discern patterns that signify economic expansions or contractions. For example, if GDP figures begin to consistently rise above a certain moving average, it may indicate strengthening economic conditions.
This method is beneficial because it allows economists to set aside outliers and focus on broader trends. Its unique feature is the ability to cannibalize complex economic data into actionable insights, making it influential at both the macro and micro levels. Yet, the reliance on historical data can introduce delays in recognizing changes, necessitating a balanced approach that pairs these insights with real-time information.
Predictive Forecasting Techniques
Another compelling application is in predictive forecasting. Moving averages can serve as foundations for predicting future economic conditions by observing historical trends and projecting them forward. They provide a framework for understanding how various factors could influence future outcomes, which is incredibly valuable for investments and policy decisions.
The key characteristic of predictive forecasting using moving averages is its reliance on probability. While it doesn’t guarantee accuracy, it builds on historical performance to predict potential future behavior. A pitfall of this approach is the possibility of over-reliance, where stakeholders might overlook other indicators that could provide a fuller picture of future performance.
Role in Risk Management
Identifying Volatility
Identifying volatility in markets is another strategic use of moving averages. By applying these calculations, investors can measure fluctuations in asset prices or market indices over time, providing insight into stability or turbulence. A high volatility scenario may prompt traders to adjust their positions or protect themselves against potential losses.
The unique aspect of using moving averages for volatility identification is their adaptability; they can be customized based on the sensitivity of the investor to price swings. However, while they can reveal trends, they might lag when sudden market movements occur, which can affect the decisions made at critical moments.
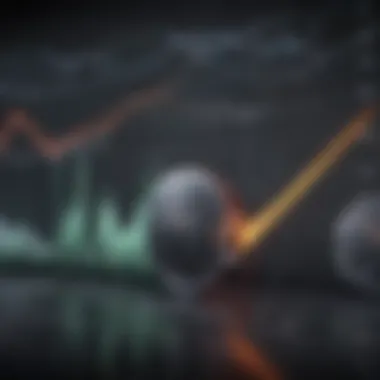
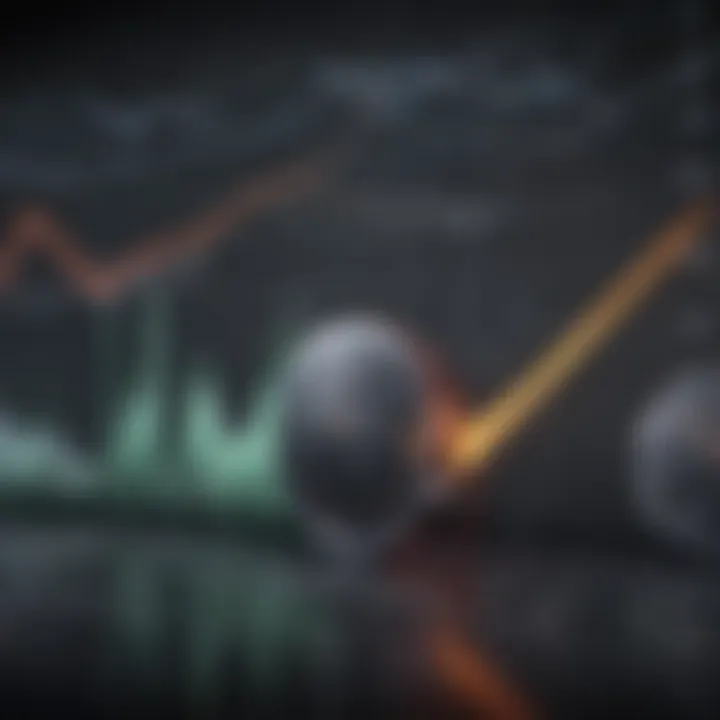
Risk Mitigation Strategies
Finally, moving averages can be integral to developing effective risk mitigation strategies. By incorporating moving averages into trading strategies, investors can set stop-loss orders based on the movements of those averages. This measure ensures that even in the face of volatile market conditions, underlying risks are well managed, preserving capital and maintaining trading discipline.
The defining feature of this approach is its proactive nature; rather than reacting post-event, traders can set parameters in advance. Still, it’s important to remember that relying entirely on moving averages can sometimes obscure insights from other analytical tools and market indicators, which could leave traders vulnerable to unseen risks.
Common Pitfalls in Moving Average Calculations
When it comes to calculating moving averages, even the most seasoned analysts and investors can stumble upon a few common pitfalls. Recognizing these missteps is paramount to ensuring that your calculations and subsequent interpretations align with the underlying data trends. By understanding these issues, you can significantly enhance the reliability of your analyses. Here, we discuss three main pitfalls: overfitting data, ignoring market conditions, and misinterpreting signals.
Overfitting Data
Overfitting, in the context of moving averages, refers to the practice of tailoring a model too closely to historical data. This can lead to a false sense of accuracy in predicting future trends. Investors may be tempted to use overly complicated or numerous moving averages, convinced that this approach will yield better insights. However, this often results in a model that reacts too sensitively to minor fluctuations in the data, rather than capturing the essential trends.
"A model that fits every wiggle in the historical data is like a GPS that gets you lost but insists it has the best route!"
In practical terms, overfitting can lead to:
- Complicated strategies that require excessive monitoring and adjustment.
- False signals that dismiss potential opportunities when the market doesn't behave as anticipated.
Thus, it's important to strike a balance between simplicity and complexity in your moving average approach. Focus on the most relevant moving averages that reflect true market behavior rather than getting lost in a sea of overly tailored data.
Ignoring Market Conditions
Market conditions can fluctuate dramatically based on a host of factors—economic changes, geopolitical events, or even natural disasters. Ignoring these conditions when calculating and interpreting moving averages can lead to misguided decisions.
For instance, during a bull market, a simple moving average might give the illusion that a particular asset is persistently gaining value. However, if the economy shifts towards recession, the same moving average can mask underlying weaknesses.
Consider this:
- In cyclical markets, moving averages can provide distorted signals if not contextualized with broader market trends.
- Ignoring external news and events can render your moving average calculations nearly useless.
In essence, appreciating the environment in which you're operating adds a layer of nuance to your analyses. By integrating broader context, you can avoid the trap of believing that past performance is truly indicative of future results.
Misinterpreting Signals
Misinterpretation of moving average signals is another pitfall that can cost investors dearly. Different types of moving averages (simple, weighted, exponential) provide varying signals based on their calculation methods. This can lead to confusion, especially for those who are new to using these tools in analysis.
For instance, a trader might misread a crossover signal where a short-term moving average crosses below a long-term moving average as a clear sell signal. However, if market conditions favor an upward reversal soon after the crossover, the trader could end up losing out on potential gains.
Key points to consider:
- Understand the specific characteristics of each moving average type to ensure proper signal interpretation.
- Heightened volatility might require different interpretation of signals than when the market is stable.
In closing, make sure to always cross-reference moving average signals with additional analysis tools and market conditions. This layered approach helps create a more robust decision-making framework, ensuring you stay one step ahead in the game.
The End
In wrapping things up, the importance of mastering moving averages cannot be overstated. This statistical tool serves as a lighthouse in the often turbulent waters of data analysis. By understanding how to calculate and interpret moving averages, individuals can make more informed investment decisions, gauge market trends, and even forecast potential economic shifts. The knowledge of differing types of moving averages—simple, weighted, and exponential—equips investors with the flexibility to adapt their strategies according to specific market conditions and their own financial goals.
One of the key elements discussed throughout this guide is the versatility of moving averages across various disciplines. This adaptability enhances their relevance, as moving averages can serve different purposes, from analyzing stock movements to assessing macroeconomic indicators. Navigating the nuances of each type facilitates a more comprehensive understanding of data, promoting a strategic approach to managing investments. Investing time into this foundational technique bears substantial fruits, notably in risk management and decision-making processes.
Thus, to recap the core benefits:
- Clarity in Market Trends: Moving averages smooth out price fluctuations, granting a clearer picture of underlying trends.
- Informed Investment Strategies: Tailoring approaches with different moving averages can lead to more savvy decisions.
- Risk Mitigation: Recognizing patterns helps in understanding potential volatility, allowing for preemptive measures.
As financial landscapes evolve, staying current with these techniques is crucial for both novice and seasoned investors alike.
Recap of Key Takeaways
To effectively draw insights from our discussion on moving averages, let’s crystallize some of the main takeaways into concise points:
- Understanding the definition and importance of moving averages aids in various analytical tasks.
- The three major types of moving averages—simple, weighted, and exponential—each serve unique purposes and contexts.
- Calculating moving averages involves processes that can be tailored for specific data sets, enhancing the relevance of results.
- Practical applications underscore their role across sectors from finance to economics, demonstrating their utility in real-world scenarios.
- Common pitfalls are plentiful; being aware of them paves the way to avoid costly mistakes.
Future of Moving Averages in Data Analysis
Looking ahead, the landscape for moving averages in data analysis is poised for significant transformation. As technology continues to advance, the integration of artificial intelligence and machine learning into financial analysis may lead to a more nuanced application of moving averages. This means we could see smarter algorithms that can predict market anomalies based on complex moving average computations.
Also, with the explosion of big data, the ability to analyze vast streams of information combined with moving average techniques will undoubtedly refine forecasting capabilities. Investors will not just look at static numbers; rather, they’ll analyze trends derived from real-time data, lending more agility to trading strategies.